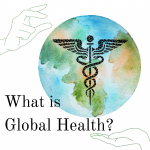
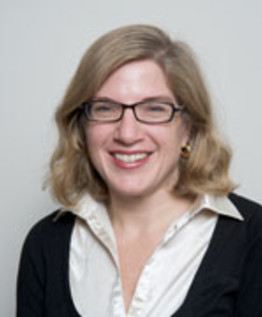
In this episode of “What is Global Health,” Monica Manmadkar spoke with Dr. Mary Beth Terry from Columbia University’s Mailman School of Public Health on the malaria epidemic. Dr. Terry gives insight into how data science is being used increasingly in public health analyses and research.
Dr. Terry is a professor at the Mailman School of Public Health at Columbia University Medical Center. Her research focuses on cancer prevention with a specific focus on breast cancer. She is a cancer epidemiologist with over 20 years of leading studies of cancer etiology specifically focused on the role of genetics, epigenetics, and other biomarkers play in modifying the effects of environmental exposures.
Dr. Terry currently leads NIH grants funded through the National Cancer Institute and the National Institute for Environmental Health Sciences that focus on following cancer risk within family-based cohorts focused on studying environmental exposures during key windows of breast cancer susceptibility. She is also leading prospective studies to validate and extend breast cancer risk assessment models. She is also funded through the Breast Cancer Research Foundation.
Dr. Terry has authored or co-authored over 300 scientific publications. Her more recent work supports that environmental exposures and biomarkers are associated with modifying risk even within high risk families. Her work also focuses on measuring risk factors for mammographic density, a strong intermediate marker of breast cancer. In addition to her doctorate in epidemiology, Dr. Terry has a Master’s degree in economics and previously worked as an econometrician and program evaluator for a number of government-sponsored programs. Dr. Terry teaches introductory and advanced epidemiologic methods.
Transcript (Via Sonix)
Monica Manmadkar: [00:00:13] Hello and welcome to the next episode of What is Global Health, a student run podcast series by the Journal of Global Health at Columbia University. In this series, we interview experts in the field to learn more about topics ranging from COVID 19 to menstrual health and hygiene. We aim to factor all elements of identity, race, gender, sexuality, religion and more into these discussions of global health. My name is Monica Manmadkar. I’m currently a sophomore majoring in computational biology. I’ve got to speak with Dr. Mary Beth Terry about data science and its usage in public health. Let’s get started.
Dr. Mary Beth: [00:00:52] Thank you so much, Monica, and please feel free to call me. Mary Beth. I’m a professor of epidemiology and environmental science in the School of Public Health, as you say, and I am super excited to be here with you and talk about data science and specifically our mission of increasing diversity in the data science workspace. So that was one of the reasons we put together this proposal that has been funded through the Data Science Institute for the last two years.
Monica Manmadkar: [00:01:22] Definitely. So, I guess my first question to you would we would be before we kind of delve into the data science aspect of it is you got your PhD from Columbia University, you got your bachelors from George Washington and your master’s from a University of Washington. Could you tell me a little bit about like how throughout your education and getting your master’s and PhD, did you develop your interest within like public health?
Dr. Mary Beth Terry: [00:01:49] Sure. I actually I got involved in public health through probably a nonlinear pathway, which is true for a lot of people in public health. So, my background prior to going into epidemiology specifically was in econometrics and economics. So, I was doing similar modeling that I do now, but specifically using data sets like from the Department of Labor, or we did some work on cost effectiveness of different health care plans. And it was really the work in the latter that I was doing long time ago. So, way before you were born in the early 1990s, I was working, doing a lot of cost effectiveness of different kinds of medical plans, and while I was doing that, I got exposed to epidemiology by reading some of the articles I needed to do to do those research projects. And I really liked the field. And so, I got involved in working on different epidemiological projects and then decided I wanted to get my PhD in that.
Monica Manmadkar: [00:02:50] That’s super, super great. I mean, you went from like international affairs and economics into like epidemiology, and now you’re working on the intersection between data science and epidemiology and public health, which I think is super, super interesting and really a great way. I mean, it also goes to show that like you can always get involved in this vast field of global health and public health, even if like get your bachelor’s there, even if your master’s is not like directly related towards the major. And there’s just so many ways to get involved. Um, so I guess my next question to you would be like, how do you think that data science has been making an impact in terms of like the public health sphere and, and in other spheres as well in terms of like health care and medicine?
Dr. Mary Beth Terry: [00:03:40] That’s a great question, Monica. Thank you. Yeah. So, I guess perhaps it’s important to think about what is data science, right? So, data science is really a lot of different areas. Some people say, you know it when you see it, it includes kind of more of the traditional quantitative sciences, at least in public health and medicine, including obviously biostatistics and epidemiological kinds of analyses. But the larger field of data science that’s also relevant to medicine and public health has been the increasing use of, you know, artificial intelligence and AI based deep learning methods, specifically in developing clinical algorithms. And that probably was the main reason I really when I was approached by my partner teaching this class, Dr. Greenleaf, who’s a demographer, um, I was approached to do this with her and that was one of the reasons I signed on because really the increased use of clinical algorithms in medicine and public health has really, you know, grown exponentially, so to speak, because really the electronic health record and lots of other kinds of data sources are readily available for large data analyses, more so than ever before. But the people really creating a lot of these algorithms, you know, for the most part until recently have been, you know, fairly homogeneous. And so, I do really think that the best algorithms are really developed by very diverse groups of people that take in the lived experience, understand all the different kinds of macro and micro determinants of health. And so, I really wanted to teach this class to get more people excited about data science and programming specifically.
Monica Manmadkar: [00:05:37] Definitely. I think there’s definitely like a lot of I guess even from my perspective, a lot more applications that I’ve seen of data science within medicine and within other aspects of other fields as well that I can see from my friends and my other peers. And I think something that we touched upon that you guys have touched upon in lectures, and I’d love to bring that topic into the podcast as well, is how there are certain issues with big data and especially when it comes to health care and health related data that is more private and more secure. What would you say are some of the with all the benefits? On one hand, that big data and its analysis can offer the public health field? What are maybe some cons of these type of analyses?
Dr. Mary Beth Terry: [00:06:23] Sure. Another great question. So, I guess maybe let’s start with the big vision of all of this is that, you know, data can lead to more accurate application of population health. So more accurate applications of programs that can be effective in the community for prevention programs that can really kind of address in a major way through data and through revealing major health inequities. That’s the promise, right? From everything from precision medicine where people are not overtreated but they’re not undertreated, and that the data themselves can reveal really where most of the focus needs to go. Now, the con, as you say, can be very great as with anything, right? So again, the con can be if you know, as we live in an algorithmic world, more and more and this is not true only for health and medicine, but it’s true for all sorts of disciplines and fields, is that those algorithms are developed and trained on the data that they have. And again, not understanding maybe what goes into those algorithms, then, you know, people can be given the false kind of may have the false belief that those are fair algorithms. And so that’s why I think it’s super important to have a really diverse group of people, you know, unpacking those algorithms, understanding what are the drivers of health and really understanding when they can be used for good and when they can be misused.
Monica Manmadkar: [00:08:04] Mhm. Definitely. I think that’s, that’s a really important point and a really great point at that, especially pinpointing at the usage of data and how, when and where it could be used. Um, can you talk maybe a little bit about kind of going back to your background and how you got into the field? Can you talk maybe a little bit about some of the research that you have conducted within like the intersection of data science and public health and how you have maybe advanced your knowledge or your or your breath in in this intersectionality of these two fields.
Dr. Mary Beth Terry: [00:08:38] Sure. Maybe. Again, just because I’ve been around a little while, I can tell you kind of the arc of how much things have changed for studying certain things. So right now, there’s a huge potential to learn more and more about how environmental chemicals can influence our health. So, in the past, the primary way to do this, at least for cancer, which is one of the areas that I focus a lot in, really had been from these very large occupational cohorts. And so, people who we know are exposed for many years based on their job titles and those have revealed very important chemicals that, you know, can affect your risk of cancer as well as other health outcomes. But, you know, the other part of this is for people who are not in these occupations but are exposed to very common chemicals every single day, the air that we breathe, the water that we drink, the food that we eat, all of those kinds of sources are very hard in epidemiology to really, you know, to really accurately describe. So, it’s very hard to do by questionnaire. And so, when I was studying for my PhD, one of the ways that was fairly new at the time was to look at measures of these kind of environmental exposures through blood or urine. And that’s still being used. But now because of, you know, big data and data science, a lot of historical measurements of environmental exposure, we can capture through linkage to these very large databases, for example, from the Environmental Protection Agency or other kinds of large government data sources that just never existed before. So, data science can allow you to look at these kinds of exposures in a way that you never were able to before in order to study whether or not they’re affecting health.
Monica Manmadkar: [00:10:41] Definitely. I think I think now, especially looking into the environmental concerns of things with the Arctic. And I guess I took a when I when I took intro bio last year, I learned a lot about the ecology and how human interactions are affecting our environment, but also affecting our own human health. And I think that’s a super innovative way to look at these types of situations. Um, I was wondering if you could talk a little bit about some of, I guess, specific uses of data science within public health that you’ve seen that have, I guess, made an impact directly within the community, like something that we talked about during lecture, remember, was the opioid crisis and how looking at big looking at the data and understanding how the different trends within the different boroughs of New York made a difference and how that kind of played a part in understand the different policies and action items that we could that other policymakers and professionals outlined. What are what is maybe like one example that you could think of that had something like a like a go through impact in terms of how data science impacted the way that professionals viewed certain epidemics or crises or issues at hand.
Dr. Mary Beth Terry: [00:12:04] Um, great. Yeah, another great question. So, at its heart, epidemiology really has, you know, very much been driven by data. Now, you know, years ago that would be everything from like just looking at a spot map, for example, and tracing cases and then what happened with the spread of disease like that. Now those sorts of things are still done very much with programs that do this kind of interactive mapping. We’ll be doing mapping in a few weeks in class. And, you know, more and more what’s been shown over time is just how important mapping is to understanding public health, whether that’s from infectious disease, which certainly it’s critical for. And some of my colleagues have been behind those New York City maps of COVID, particularly a close colleague, Wang Yang, has developed a whole lot of modeling to understand the spread of COVID based on population density in neighborhoods, but chronic diseases to very much mapped to neighborhood. So, the importance of neighborhood really is critical for understanding many different diseases all throughout life. And that has become more and more apparent. As you know, the data get collected on a much broader scale around very diverse communities, you know, around New York City, around the country, around the globe. So, yeah, the ability to make these kinds of connections really has come through the availability of these public data sets.
Monica Manmadkar: [00:13:45] Definitely. Yeah. I think I think that’s a really, really great point. And kind of going off of that, um, I was wondering, looking to the future now with data science and AI and machine learning becoming ever more. Changing and evermore, I guess, evolving techniques and technologies which can be widely applied within several fields. Medicine and global health being one of them. What would you think are maybe some future applications that you can see of data science impacting the way that we look at public health and we look at the data that we are given within maybe a certain area or a certain issue or a certain public health dilemma that we that we would be facing.
Dr. Mary Beth Terry: [00:14:33] Yeah. No, I think that’s a fantastic question. I very much I’m optimistic that the more and more people who are exposed to the data and have data publicly available and have discussions about data and look at how the data were collected and the pros and cons of data, I very much feel that that can help move public health forward. So, in particular, one of the major areas in the past that I think has stymied public health has been sometimes in the way we communicate findings. You know, in the past it was more common to have kind of what they call this hierarchical deficit model where, you know, the public health rules and recommendations are given from up high down to the community. The reality is that for public health and for community health, we need to engage much more in a dialogue model where all the stakeholders are involved in discussing what’s relevant, what research should be done in the community, and where everyone has access to the data and is empowered to use the data to understand ways to improve health. So, I very much think that the more we can engage people with the data and have, you know, very transparent, um, uh, ways of, of making that data available to everyone and collecting the data in a very bidirectional way so that all parties are part of the whole process. The more and more whatever data that get evaluated and analyzed, then that will be more impactful than, than to start without that kind of conversation from the beginning.
Monica Manmadkar: [00:16:31] Definitely. I think I think I’m personally as a computational bio major, I’m really, really excited to see where the field goes in the next ten, 15, 20 years, um, and how it, how it grows over time and how the way we look at and how we approach a lot of these situations change, I guess, given the pandemic and given like everything that happened in the past two years, it’s really important to kind of look at, I guess, that dialogue based conversations with all the stakeholders involved is something that I think everyone is now personally aware of because of how the policies masking policies and how that may be affected by public health data and how people need to like how vaccines and how all these different things like different factors all kind of come together in this in this very intersecting, um, intersecting manner. I think my next question to you would be that, I guess kind of taking it back a couple steps, how do you think that data science has helped or could have maybe helped more to understand the pandemic and understand COVID 19, something that I guess has been an awakening for many people in terms of understanding the public health realm?
Dr. Mary Beth Terry: [00:17:54] Um, yeah, no, that’s a great question. I think that, uh, data science has been critical at every stage of the pandemic, and the models were critical in terms of informing policies, particularly in New York City, in terms of, um, you know, our even within Columbia, our own institution, the institutional policies, all of those were data driven. I do think that, um, you know, data science and health communication are, um, are tied together very closely and so and both need to be grounded in, you know, science education. So, I think, um, uh. Making data science methods and data science kinds of making it more accessible to everyone at every level. And that the more and more we do that, I think the better we can be in terms of coming to some sort of agreement rather than having data science be this mysterious thing that is thought to be, you know, only one thing. And it’s really applied to every field that that that that we have. And that’s why we believe designing kind of the course around an application which is doing you know a New York City Department of health data brief would make people interested in learning our programming who might never be interested in learning programming for just learning programming’s sake. So, I do think the more we make any of these kinds of methods accessible so that people can use them, you know, and apply them within their own settings so that they will then be at the table when these policy decisions are being made. I think that will help a lot.
Monica Manmadkar: [00:19:57] Definitely. Yeah, I think I think that’s a great point. And I guess wrapping it up now with my last question to you would be what would your advice be for students who are looking to get involved in in this field of data science and public health and to get there to get a better understanding of how computational methods and statistical analyses can be increasingly used to analyze global health issues. What would your advice to those students be in terms of what they should maybe do or what resources they should maybe take advantage of or how they should maybe approach these types of, I guess, issues?
Dr. Mary Beth Terry: [00:20:40] Yeah. No, that’s a great question. I think there are many pathways to improving health. I don’t think it just has to be quantitative science. And there’s so much important, um, uh, important work that’s being done in qualitative science so it’s more to know enough that you can be part of the conversation. Most science, the best science is always interdisciplinary with large teams of people because that’s where you get the most large teams, but large, diverse teams, diverse in terms of life experience, diverse in terms of discipline. The more you can get people around the table that are different from one another, the better. Whatever kind of health policy or risk algorithm you develop and try to validate the more generalizable and more impactful it will be. So, I think if people are interested in public health, well, certainly on either the Barnard or Columbia campus, there’s lots of opportunities. We have not only just multiple schools of public health within New York City, but we have the oldest and largest department of health in the nation. So, there’s a lot of opportunities right here in New York. And then, you know, again, I think the value of always being a student, I know that might not sound fun for me for being a student right now, but like I do feel like that’s where, you know, a data science and methods will always evolve. But if you feel kind of grounded in the principles of quantitative science and statistics and other kinds of principles, then, you know, however it evolves and the data sources, you’ll be in a good place to critique things in a very analytical way.
Monica Manmadkar: [00:22:27] Definitely. I think I think that’s a that’s a great point that you bring up, that people can get involved in both the quantitative and the qualitative methods and just learning about the opportunities available here at Barnard and at Columbia within both of the camp campuses and think that’s all from me. And thank you so much, Mary Beth, for taking the time to interview. It’s been a great pleasure talking to you and learning more about the intersectionality of data science and public health and how its applications, whether it be future or present or in the past with the pandemic involved, involved. I think it’s been a great opportunity to learn more and for our listeners as well. Thanks for tuning in to this installment of What is Global Health. We hope you enjoyed and as always, be on the lookout for new episodes every other week.